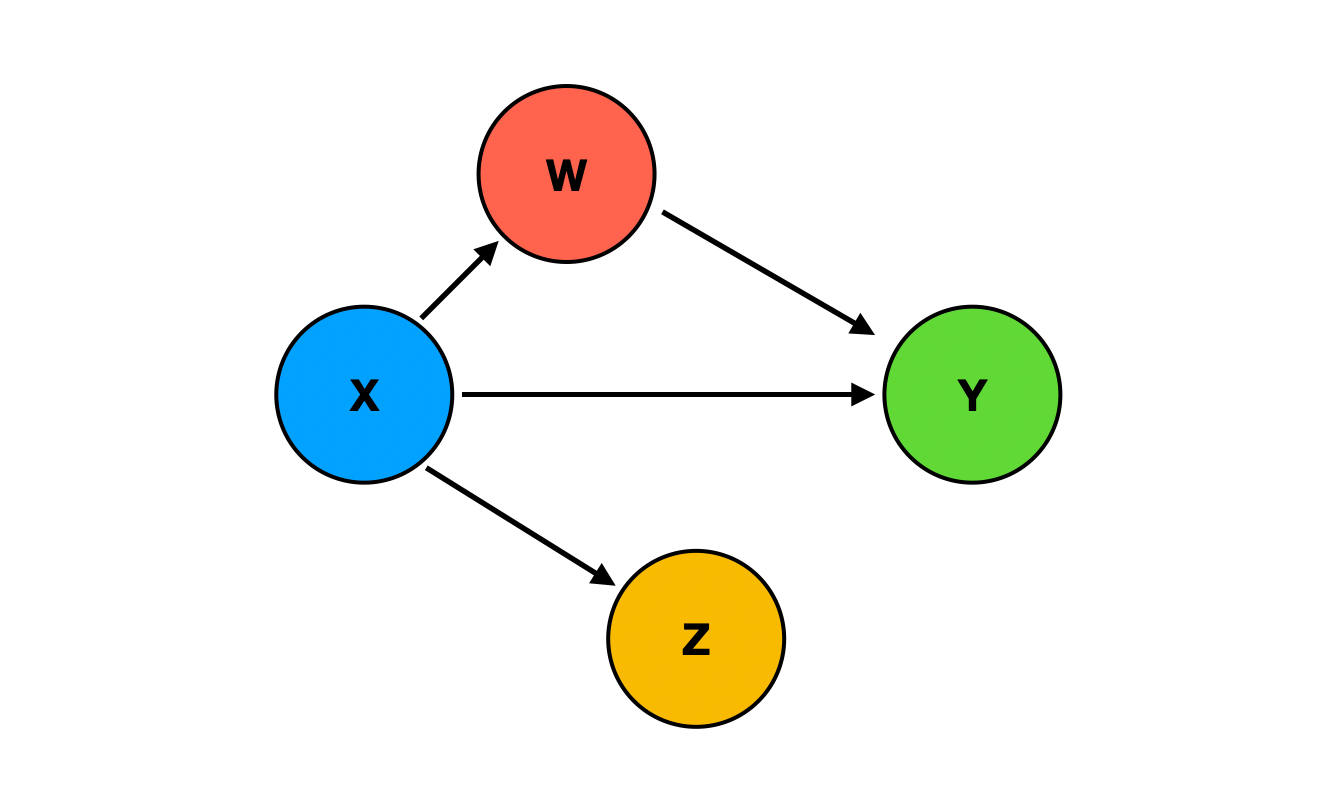
Causal inference helps us understand the cause-and-effect relationships in various fields like medicine, economics, and social sciences. But what exactly is it? Causal inference is the process of drawing conclusions about a causal connection based on the conditions of the occurrence of an effect. Imagine trying to figure out if eating more vegetables leads to better health. You'd need to consider many factors, right? That's where causal inference comes in, using methods like randomized controlled trials and observational studies to untangle these complex relationships. By understanding causal inference, we can make better decisions, improve policies, and enhance our understanding of the world around us. Ready to dive into some intriguing facts? Let's get started!
What is Causal Inference?
Causal inference is the process of determining whether one thing causes another. It's a crucial concept in fields like statistics, epidemiology, and social sciences. Understanding causal relationships helps in making informed decisions and predictions.
- Causal inference involves identifying cause-and-effect relationships between variables.
- It uses statistical methods to estimate the impact of one variable on another.
- Randomized controlled trials (RCTs) are considered the gold standard for causal inference.
- Observational studies can also be used but require more complex statistical techniques.
- The counterfactual approach is a key concept, imagining what would happen if the cause did not occur.
Why is Causal Inference Important?
Understanding causal relationships can help in various domains, from medicine to economics. Knowing what causes what can lead to better policies, treatments, and interventions.
- In medicine, causal inference helps determine the effectiveness of treatments.
- In economics, it aids in understanding the impact of policies on economic outcomes.
- It can help identify risk factors for diseases and conditions.
- In education, it can assess the effectiveness of different teaching methods.
- Businesses use causal inference to understand the impact of marketing strategies.
Methods of Causal Inference
There are several methods to determine causality, each with its strengths and weaknesses. Choosing the right method depends on the context and available data.
- Randomized controlled trials (RCTs) are the most reliable but can be expensive and unethical in some cases.
- Natural experiments use real-world events to study causal effects.
- Instrumental variables help address issues of confounding in observational studies.
- Difference-in-differences compares changes over time between a treatment group and a control group.
- Propensity score matching attempts to mimic randomization by matching treated and untreated units with similar characteristics.
Challenges in Causal Inference
Determining causality is not always straightforward. Various challenges can complicate the process, making it essential to use robust methods and careful interpretation.
- Confounding variables can obscure the true causal relationship.
- Selection bias occurs when the sample is not representative of the population.
- Measurement error can lead to incorrect conclusions about causality.
- Reverse causality happens when the direction of cause and effect is unclear.
Final Thoughts on Causal Inference
Causal inference is a powerful tool for understanding the relationships between variables. By distinguishing correlation from causation, it helps researchers make more accurate predictions and informed decisions. Techniques like randomized controlled trials, natural experiments, and instrumental variables are essential for establishing causality.
Understanding these methods can improve the quality of research in fields like medicine, economics, and social sciences. While challenging, mastering causal inference offers significant rewards, including better policy-making and more effective interventions.
Keep in mind, though, that no method is foolproof. Always consider the context and limitations of your data. With practice and careful application, causal inference can become a valuable part of your analytical toolkit.
Was this page helpful?
Our commitment to delivering trustworthy and engaging content is at the heart of what we do. Each fact on our site is contributed by real users like you, bringing a wealth of diverse insights and information. To ensure the highest standards of accuracy and reliability, our dedicated editors meticulously review each submission. This process guarantees that the facts we share are not only fascinating but also credible. Trust in our commitment to quality and authenticity as you explore and learn with us.